Nutrition 2.0: Analyzing your biochemical individuality with mathematical precision to serve up your ideal diet
Today’s nutritional regime, which we call Nutrition 1.0, is inadequate to address your biology and your specific health issues, for many reasons. Nutrition 2.0 is a new approach to nutritional science that uses your molecular data (chemistry) and advanced algorithms (math) to compute the optimal nutrition for you individually1 2. The goal of Nutrition 2.0 is to understand the specific molecular processes in your biology, so that your nutrition can be computed to maintain healthy functions in your entire body. Our AI-generated nutritional recommendations are highly personalized and custom-crafted for your biology. This is radically different from one-size-fits-all diets like ketogenic or paleo.
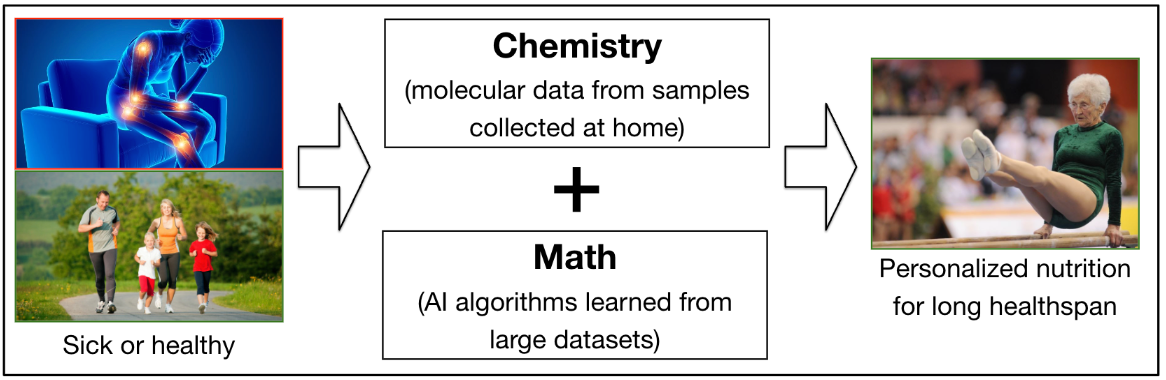
The main features of Nutrition 2.0 platform are:
It is 100% data-driven and objective (chemistry + math). There are no humans involved in the process from the moment samples enter the lab until the results and recommendations are delivered to the user.
It is highly personalized. There are as many diets as there are users. There are no fad diets, and it prioritizes customized over generic recommendations (such as “eat more fiber” or “decrease sugar intake”).
Its goal is to prevent/cure chronic diseases and cancers, and slow down aging.
It is continuously learning and improving, as it uses all the platform data to improve its algorithms. Therefore, its accuracy is getting better every day.
We start with the molecular data obtained from your biological samples collected at home non-invasively, and information collected from questionnaires on your smartphone app. We then convert them to nutritional recommendations using clinically validated laboratory tests and advanced mathematical algorithms rigorously designed and validated by world-class experts for over a decade. Your precise nutritional recommendations are determined in a fully data-driven and automated manner using these validated chemical and mathematical methods. There is no need for human decision making, since the decisions have been validated and incorporated into the system ahead of time – thus this system is highly scalable to every individual on the planet. Moreover, a Nutrition 2.0 system is continuously learning and always improving, because all available new data is repeatedly analyzed and re-validated. As the number of people who follow Nutrition 2.0 increases, it also improves the accuracy of the platform.
Nutrition 2.0 is a quantum leap from the current nutritional practices that have severe limitations, such as heavy reliance on population studies that treat all humans as similar (thus only consider the ‘average’, ignoring biological individuality), nutritionists’ experience and beliefs, and fad diets that are marketed without evidence to be good for everyone (ketogenic, etc.). The Nutrition 2.0 concept has been implemented within the Viome system, and has been shown to work effectively in a number of studies.
Today’s nutritional practices do not account for your biochemical individuality
Your life story is unique to you, and so is your biology. Yes, your DNA provides a starting point, but the current state of your biochemistry is largely driven by your genetic expression (RNA) based on your nutritional history, environment, and lifestyle. As you write the next chapters of your life story, you have many choices, and you want to make it the best possible story. Unfortunately, today’s Nutrition 1.0 does not help you with that.
Say you have a set of symptoms and present those to three different nutritionists, you will likely receive three different nutritional recommendations when it comes to specific foods. This should not be how a scientific method works – what would you think of mathematics if you asked three mathematicians “what is 2+2”, and you received three different answers?
In today’s Nutrition 1.0, each nutritionist has a different background and experience. More importantly, their recommendations may not accurately reflect your individual biology. The most egregious examples are the recommendations to follow fad diets, promoted by some influencers and bloggers as good for everyone and every condition. If such a diet existed, there would be very strong scientific and clinical evidence for it, but that is not the case. Asking everyone to follow a fad diet is like asking everyone to play the same sport, or have the same hobby, which is not the best solution for all.
While there is strong epidemiological and molecular evidence that the human diet contributes significantly to the onset and progression of many chronic diseases and cancers, dietary advice has been controversial and polarized. Scientific publications often contradict one another, with one demonstrating health benefits while another suggests potential harm for the same foods or diets. Here are some of the many examples:
A large epidemiological study 3 reports that daily consumption of fruit juice raises cancer rates significantly. Other studies report that the consumption of daily fruit or fruit juice is healthy 4 5.
Dairy consumption has generated contradictory claims, with some evidence supporting an association with a higher incidence of some cancers and chronic diseases, some studies showing no such correlations, while other studies demonstrate significant benefits 6 7 8.
Likewise, recent publications provide conflicting data on the effects of saturated fats on cardiovascular and metabolic diseases 9 10.
Red meats have been touted as healthy sources of critical nutrients, such as vitamin B12 and dietary protein, and even shown to provide health benefits; yet there is strong epidemiological and mechanistic evidence that red meat may be responsible for increased rates of inflammatory diseases and cancers 11 12 13.
To complicate the situation further, current food and supplement guidelines are influenced by the food industry, which promotes the increased consumption of sugars, highly processed foods, dairy, and meat. The large body of conflicting food and supplement information has manifested in the creation of hundreds of diets, each claiming health benefits. When it comes to scientific data, the vast majority of food and supplement research does not account for the specific and unique contributions of an individual’s gut microbiome, despite lots of evidence showing that the impact is significant. The USDA-provided nutritional guidelines are the same for everyone and based on population averages. These guidelines do not consider personal preferences, food intolerances or food allergies either.
Very significantly, there is recent strong scientific evidence that human genetics plays a minor role, and the gut microbiome plays a major role in the effects of nutrition on human health 14 15. This was demonstrated in a large twins study of metabolic disease parameters 16. This study observed large inter-individual variability in postprandial blood triglyceride, glucose, and insulin responses to identical meals. It is clear that the gut microbiome not only influences how food is digested, but also converts many of the molecular ingredients found in foods into beneficial or harmful secondary metabolites that have profound effects on human physiological functions, such as neurotransmitter production, immune system activation and deactivation (inflammation), immune tolerance, and carbohydrate metabolism.
Viome’s Nutrition 2.0 platform
Viome has developed a data-driven approach to personalized food and supplement recommendations that is powered by artificial intelligence and individualized molecular data from the human microbiome, the human host (you), and their interactions. The process starts with a clinically validated transcriptomic analysis of a person’s stool, blood, and/or saliva samples17 18 19. This method measures the biochemical functions (“chemistry”), of all microorganisms, their genes, and human genes, interacting together as one super-organism to maintain human health. These chemical data, combined with the survey data, are converted into personalized nutritional recommendations (foods and supplements) using mathematical algorithms (“math”) derived from the vast amounts of data that Viome has gathered and continues to generate. The algorithms use machine learning, as well as logic, that was developed from clinical research studies and domain knowledge1. This process is described in more detail in another blog.
Technological advances in genomics and computational biology have made it possible for food and supplement science to adopt a paradigm shift. Each food can now be viewed as a container of molecular ingredients (i.e. micronutrients), rather than a homogenous material that is either good or bad for human health. Most nutritional guidance is offered for generic food groups, nutrients, herbs, spices or even supplements. However nutritional science is moving in a precision-driven way. Hence, the metabolic functions of each person’s unique microbiome need to be identified and quantified, in order to “prescribe'' specific foods that contain micronutrients that will be converted to beneficial secondary metabolites by that person’s individual gut microbiome. Microbial functions also need to be quantified so each person can avoid foods that contain micronutrients that will be converted by their gut microbiome into harmful secondary metabolites that are associated with disease.
The figure below shows a high-level overview of Viome’s food recommendation system that incorporates the above ideas. A food ontology with precise information about each food staple is curated. The macronutrients from each food is used to precisely determine the glycemic response of the food within each individual, via a machine learning algorithm 27. The micronutrients from each food are evaluated in the context of a collection of pathway activities, such as butyrate production, oxalate elimination, sulfide production, etc, each of which is computed using the individual’s microbiome as described in another blog. All of this information is used to determine whether each food is a superfood, enjoy food, minimize food, or avoid food for the specific individual. A similar method is used for supplement ingredients as well.
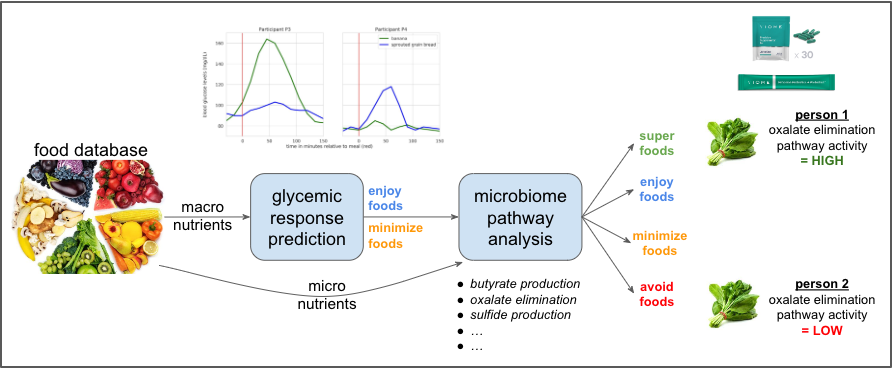
In addition to the approaches that utilize known microbial metabolites and their micronutrient precursors, machine-learnt models from large clinical studies need to be applied to each person’s gut microbiome analysis to guide the best choice of foods and supplements. Such models have already been developed for personalizing foods rich in carbohydrates20. These data-driven food choices can minimize the blood glucose levels. Besides the human microbiome, an analysis of human gene expression must be integrated into the systems biology view of the human body to enable the understanding of the network interactions among the dietary molecular ingredients, the microbiome, and human physiology. This understanding is critical because human gene expression, whether modulated by genetic, food and supplement, microbial, or other environmental factors, has a direct connection with the onset and progression of chronic diseases.
Does Nutrition 2.0 work?
We have preliminary data that Nutrition 2.0 can reduce the signs and symptoms of several gastrointestinal and mood conditions. These findings have been published in two pre-prints and are currently undergoing peer review 1 2.
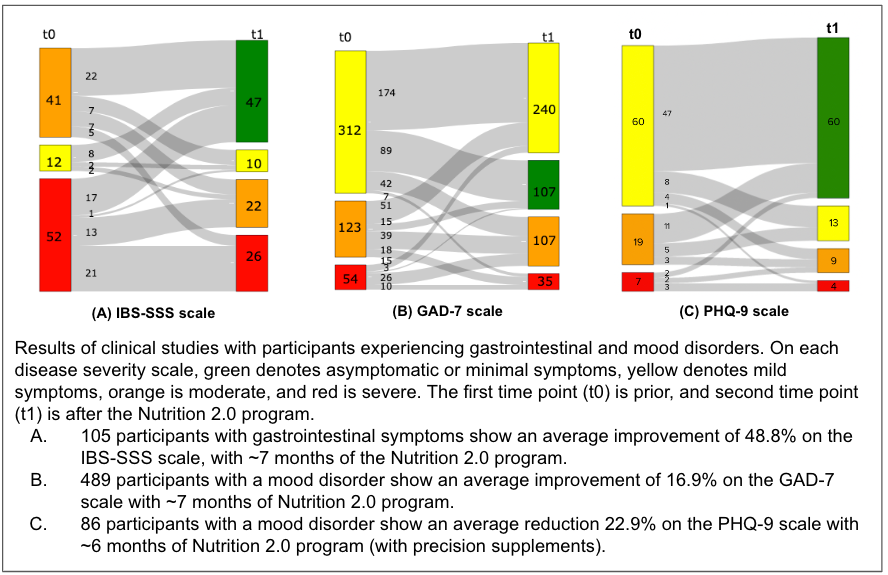
Gastrointestinal symptoms such as abdominal discomfort, diarrhea, or constipation afflict 10 to 15% of Americans21. Clinicians have developed validated questionnaires such as Rome IV and IBS-Symptom Severity Score (IBS-SSS) that are used as the gold standard for assessing gastrointestinal symptoms severity22. IBS-SSS is a composite score of abdominal discomfort, number of days with abdominal discomfort, bloating/distension, satisfaction with bowel habits, and quality of life. Each measure is rated from 0 to 100, with the total score ranging from 0 to 500, graded as mild (75–174), moderate (175–299), or severe (300–500). We performed a clinical study with more than 100 participants with mild, moderate, or severe symptoms (i.e., IBS-SSS score of at least 75) at the initial time point. After an average of ~7 months of being given the Viome Nutrition 2.0 program, we saw improvements in their IBS-SSS categories as shown in figure (A), with an average improvement of almost 40% for severe cases, and more than 50% improvement for mild and moderate cases. A 15% change in the IBS-SSS is considered to be clinically significant.
Mood disorders are highly prevalent, afflicting almost 20% of Americans. We conducted two studies on mood disorders. In the first one, we used a clinically validated GAD-7 questionnaire, with each question scoring 0, 1, 2, or 3 points, for a total maximum score of 21. A GAD-7 score of 5–9 describes mild, 10–14 moderate, and 15–21 severe symptoms23. In one of our studies, almost 500 participants with a GAD-7 score of 5 or greater were given the Viome Nutrition 2.0 program for a period of about 7 months on average. Improvements are shown in figure (B) above, with an average improvement of 16.9%. For the second mood study, we used the clinically validated questionnaire called PHQ-9, with scores ranging from 0 to 27 total. A score of more than 10 describes mild, more than 15 moderate, and more than 20 severe symptoms24. In our study, 86 participants with mild, moderate, or severe mood disorder at the initial time point were given precision supplementation as part of their Nutrition 2.0 intervention for a period of 6 months on average. Improvements after the Viome nutrition are shown in figure (C) above, with an average improvement of 22.9%.
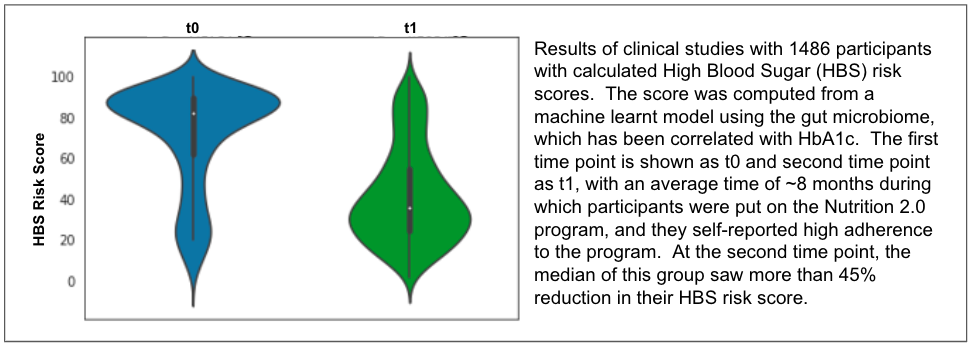
We understand that the ideal way to measure these improvements is by controlling for age, sex, length of intervention, and other factors – typically done by comparing the intervention arm with control arms. In fact, two of the above studies were controlled – the mood disorder study with a control arm without precision supplements, and the HBS study with a control arm with low adherence to the Nutrition 2.0 program. In the mood disorder study, a higher proportion of participants subscribed to the Nutrition 2.0 precision supplement program improved their symptoms (as measured by PHQ-9 categories) compared to those who did not follow the Viome food recommendations (77.3% vs. 20.8%). In the HBS study, participants adherent to the Nutrition 2.0 program improved their HBS risk score by 30% more, compared to those who did not adhere to the program. The details of these studies are beyond the scope of this blog, and are currently under review in peer reviewed journals.
In conclusion
Nutrition 2.0 is a new paradigm in nutritional sciences that uses each person’s molecular data to compute highly personalized and accurate nutritional recommendations that fine tune the person’s physiology to maintain health. It is a sophisticated platform that:
Analyzes human samples (typically stool, blood, and saliva) using cutting edge RNA sequencing (a.k.a. metatranscriptomics) to produce highly accurate data about the activity (expression) of human and microbial genes 18 19 26. This data is what we call “chemistry”, since it relates to the chemical functions of the genes.
Uses machine-learning algorithms (a.k.a. mathematical equations) that transform the chemical data into highly personalized nutritional recommendations for each person. This is what we call “math”. These transformations are objective, automated, and rely on the knowledge learned from a very large dataset and multiple large clinical research studies 27.
Viome’s Nutrition 2.0 platform is available today to everyone, at scale.
References:
1. J. Connell, R. Toma, C. Ho, N. Shen, P. Moura, Y. Cai, D. Tanton, G. Banavar, and M. Vuyisich, Biorxiv 2021.04.24.441290 (2021).
2. C. Julian, N. Shen, M. Molusky, L. Hu, V. Gopu, A. Gorakshakar, E. Patridge, G. Antoine, J. Connell, H. Keiser, U. Naidoo, M. Vuyisich, and G. Banavar, MedRxiv 2023.04.18.23288750 (2023).
3. E. Chazelas, B. Srour, E. Desmetz, E. Kesse-Guyot, C. Julia, V. Deschamps, N. Druesne-Pecollo, P. Galan, S. Hercberg, P. Latino-Martel, M. Deschasaux, and M. Touvier, Bmj 366, l2408 (2019).
4. T. A. Khan, L. Chiavaroli, A. Zurbau, and J. L. Sievenpiper, Eur J Clin Nutr 73, 1556 (2019).
5. R. Clemens, A. Drewnowski, M. G. Ferruzzi, C. D. Toner, and D. Welland, Adv Nutrition Int Rev J 6, 236S (2015).
6. G. E. Fraser, K. Jaceldo-Siegl, M. Orlich, A. Mashchak, R. Sirirat, and S. Knutsen, Int J Epidemiol 49, 1526 (2020).
7. M. Dehghan, A. Mente, S. Rangarajan, P. Sheridan, V. Mohan, R. Iqbal, R. Gupta, S. Lear, E. Wentzel-Viljoen, A. Avezum, P. Lopez-Jaramillo, P. Mony, R. P. Varma, R. Kumar, J. Chifamba, K. F. Alhabib, N. Mohammadifard, A. Oguz, F. Lanas, D. Rozanska, K. B. Bostrom, K. Yusoff, L. P. Tsolkile, A. Dans, A. Yusufali, A. Orlandini, P. Poirier, R. Khatib, B. Hu, L. Wei, L. Yin, A. Deeraili, K. Yeates, R. Yusuf, N. Ismail, D. Mozaffarian, K. Teo, S. S. Anand, and S. Yusuf, Lancet 392, 2288 (2018).
8. B. C. Melnik, Nestlé Nutrition Inst Work Ser 67, 131 (2011).
9. D. J. Ströher, M. F. de Oliveira, P. Martinez-Oliveira, B. C. Pilar, M. D. P. Cattelan, E. Rodrigues, K. Bertolin, P. B. D. Gonçalves, J. da C. E. Piccoli, and V. Manfredini, J Med Food 23, 689 (2020).
10. T. Tholstrup, C. Ehnholm, M. Jauhiainen, M. Petersen, C.-E. Høy, P. Lund, and B. Sandström, Am J Clin Nutrition 79, 564 (2004).
11. A. Astrup, F. Magkos, D. M. Bier, J. T. Brenna, M. C. de O. Otto, J. O. Hill, J. C. King, A. Mente, J. M. Ordovas, J. S. Volek, S. Yusuf, and R. M. Krauss, J. Am. Coll. Cardiol. 76, 844 (2020).
12. L. E. O’Connor, D. Paddon-Jones, A. J. Wright, and W. W. Campbell, Am J Clin Nutrition 108, 33 (2018).
13. Z. Wang, N. Bergeron, B. S. Levison, X. S. Li, S. Chiu, X. Jia, R. A. Koeth, L. Li, Y. Wu, W. H. W. Tang, R. M. Krauss, and S. L. Hazen, Eur Heart J 40, 583 (2018).
14. S. M. Rappaport, Plos One 11, e0154387 (2016).
15. R. Border, E. C. Johnson, L. M. Evans, A. Smolen, N. Berley, P. F. Sullivan, and M. C. Keller, Am J Psychiat 176, 376 (2019).
16. S. E. Berry, A. M. Valdes, D. A. Drew, F. Asnicar, M. Mazidi, J. Wolf, J. Capdevila, G. Hadjigeorgiou, R. Davies, H. A. Khatib, C. Bonnett, S. Ganesh, E. Bakker, D. Hart, M. Mangino, J. Merino, I. Linenberg, P. Wyatt, J. M. Ordovas, C. D. Gardner, L. M. Delahanty, A. T. Chan, N. Segata, P. W. Franks, and T. D. Spector, Nat Med 26, 964 (2020).
17. R. Toma, B. Pelle, N. Duval, M. M. Parks, V. Gopu, H. Tily, A. Hatch, A. Perlina, G. Banavar, and M. Vuyisich, Biorxiv 2020.05.22.110080 (2020).
18. R. Toma, Y. Cai, O. Ogundijo, L. Hu, S. Gline, D. Demusaj, N. Duval, P. Torres, F. Camacho, G. Banavar, and M. Vuyisich, Biotechniques 74, 31 (2023).
19. A. Hatch, J. Horne, R. Toma, B. L. Twibell, K. M. Somerville, B. Pelle, K. P. Canfield, M. Genkin, G. Banavar, A. Perlina, H. Messier, N. Klitgord, and M. Vuyisich, Int J Genomics 2019, 1718741 (2019).
20. H. Tily, A. Perlina, E. Patridge, S. Gline, M. Genkin, V. Gopu, H. Lindau, A. Sjue, I. Slavov, N. Klitgord, M. Vuyisich, H. Messier, and G. Banavar, Biorxiv 641019 (2020).
21. O. S. Palsson, W. Whitehead, H. Törnblom, A. D. Sperber, and M. Simren, Gastroenterology 158, 1262 (2020).
22. B. E. Lacy and N. K. Patel, J. Clin. Med. 6, 99 (2017).
23. R. L. Spitzer, K. Kroenke, J. B. W. Williams, and B. Löwe, Arch Intern Med 166, 1092 (2006).
24. K. Kroenke, R. L. Spitzer, and J. B. W. Williams, J Gen Intern Med 16, 606 (2001).
25. U. P. S. T. Force, K. W. Davidson, M. J. Barry, C. M. Mangione, M. Cabana, A. B. Caughey, E. M. Davis, K. E. Donahue, C. A. Doubeni, A. H. Krist, M. Kubik, L. Li, G. Ogedegbe, D. K. Owens, L. Pbert, M. Silverstein, J. Stevermer, C.-W. Tseng, and J. B. Wong, JAMA 326, 736 (2021).